Architecting, designing and building a robust and reliable analytics platform is an intricate process. Building a big data platform requires careful analysis and planing. Big data is part of the bigger data management architecture. It needs to satisfy not only business but also operational needs. The following section describes a high-level overview of processes and storage systems. They should be considered in on-premise or cloud environments when evaluating different analytics technologies and solutions.
Data sources
Data sources and ingestion strategies present the core foundation of an analytic platform. Some data may changes infrequently while others may need processing at higher rates. There is data which is structured while other comes in a less structured forms. Platform ingestion layer should consider and enable most of the above cases and additionally take care of fault-tolerance, data availability and the ability of horizontal scaling.
Unified stream and batch processing
Fewer and unified processing environments can result in greater developer productivity and less operational complexity. In the last few years we could see a number of new processing framework which is suitable for different use cases. When choosing the appropriate framework, we have to often make trade-off decisions. Its between performance and streaming model, delivery semantics, state management and latency requirements. Another important factor to consider is framework maturity and its application ecosystems that enables integration with other operational systems.
Operational and analytics storage layer
One of the first steps in designing a storage layer is defining technical requirements of the storage workload. The storage workload consequently drives the decision of the underlying storage approach (OLAP, OLTP, HLTP, etc.). Depending on scale and desired performance, this layer often consists of several storage and caching technologies.
Archive storage layer
Despite hardware cost reduction, big amount of data located in specialized systems like data warehouses present significant cost to the owner. One way how to reduce them is to keep rarely used data on less computational intensive but higher density storage. Such policies often enable owners to optimize their hardware cost, preserve the ability to analyze archival data or meet regulatory compliance.
Business analytics
Long-term business value of data lies in the surrounding environment of data services and their efficiency of extracting and providing insights to end users and surrounding systems. On the market, we may find a large number of BI and other analytics solutions that tries to address needs of data engineers, data scientist or data data analyst however not many of them covers all of the required functionalities. Enterprise report visualization, data discovery, advanced analytics and custom tailored solutions are just some of the options needing consideration before establishing the right analytics environment.
Previous & Next...
MapR Converged Data Platform – What Is It, And How To Use It?
MapR Converged Data Platform was MapR product. HPE acquired business assets in August 2019. MapR platform is now the integral part of HPE Ezmeral platform. What...
read more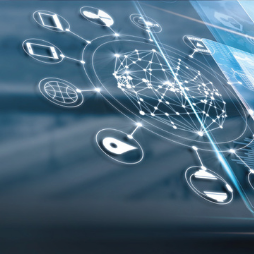
Poor Data Management Will Get You In Trouble
Data is the modern capital. Therefore, you should treat it accordingly. But – do you? I am sure no company treats its data as well as they treat their money. ...
read more