In today’s digital age, where data is a cornerstone of business strategy, the quality and governance of that data are paramount. High-quality data drives reliable insights and informed decisions, while poor data quality can lead to costly errors and missteps. This article explores the significance of data quality and governance, debunks common myths, and outlines practical steps to maintain high standards across your organization.
The Importance of Data Quality
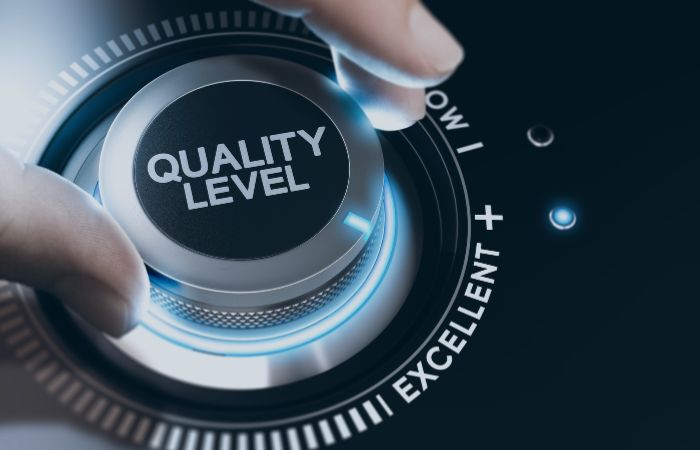
The volume of data generated every day is staggering, with estimates suggesting we produce 2.5 quintillion bytes daily. This rapid increase provides immense opportunities for businesses to make decisions based on concrete evidence rather than intuition. Companies like Amazon, Netflix, and Google exemplify the power of leveraging high-quality data for competitive advantage. However, the insights derived are only as reliable as the underlying data.
Poor data quality can have severe consequences, as seen during the COVID-19 pandemic. Inaccurate data in electronic health records (EHR) systems impeded effective patient care and public health responses, demonstrating the critical need for accurate, consistent, and reliable data. According to Gartner, poor data quality costs organisations an average of $12.8 million annually, highlighting the urgent need to prioritise data quality initiatives.
Debunking Common Myths About Data Quality
Many misconceptions surround data quality, which can hinder organisations from effectively addressing this crucial aspect:
- Data Quality is Only for Traditional Data Warehouses: Modern data environments encompass diverse sources, including IoT devices and cloud platforms. Data quality tools have evolved to handle these complexities, ensuring data integrity throughout its lifecycle. For instance, these tools use AI and machine learning to manage data from various origins and formats, such as identifying patterns in unstructured data or predicting data quality issues before they occur.
- Once Data Quality is Fixed, the Job is Done: Data quality is an ongoing process that requires continuous monitoring and updating. Changes in business processes, data sources, and regulatory requirements necessitate regular attention to maintain high standards.
- Data Quality is Solely IT’s Responsibility: Ensuring data quality is a shared responsibility across the organization. Business users, managers, and data stewards must collaborate to maintain high standards. Everyone from data scientists to application integrators should be involved, making it a company-wide priority rather than an isolated IT task. This inclusive approach makes you an integral part of the data quality team.
- Data Quality Software is Too Complex and Expensive: Today’s data quality tools are designed for ease of use, often as self-service applications. This enables non-technical users to clean and enrich data without relying on IT support. Moreover, the investment in data quality improvements is justified by preventing costly errors and enhancing decision-making and business outcomes.
Proactive Data Quality Management
Addressing data quality issues early is far more cost-effective than correcting problems later. By adopting a proactive approach and continuously monitoring and profiling data, you can ensure that any issues are detected and resolved promptly. This prudent and strategic approach embeds data quality processes throughout the data lifecycle, enhancing trust and usability.
Steps to Achieve Better Data Quality
- Observe: Continuously monitor data sources for quality and integrity.
- Discover: Profile data to understand its structure and content.
- Govern: Establish data ownership, roles, and policies.
- Standardize: Apply consistent formats and definitions across datasets.
- Consolidate: Remove redundancies and create a single source of truth.
- Operationalize: Integrate data quality practices into everyday workflows.
Choosing the Right Solution
Selecting an effective data quality solution involves several key considerations:
- Observation: Tools for monitoring quality, alerting issues, and troubleshooting pipelines.
- Discovery: Capabilities for searching, crawling, tagging, and labelling datasets.
- Governance: Functions for data modelling, lineage, and impact analysis.
- Consolidation: Techniques for data matching, merging, and creating a golden record.
- Operationalization: Features for preparing data and managing data campaigns.
- Standardization: Methods for formatting, validating, certifying, enriching, blending, and cleansing data.
A unified, cloud-based platform that facilitates collaboration between IT and business users is ideal for comprehensively managing data quality.
Conclusion
High-quality data is essential for leveraging the full potential of data-driven strategies.
Organisations that invest in data quality and governance can achieve better business outcomes, greater efficiency, and a competitive edge. With the right tools and a collaborative approach, ensuring trusted data across your organisation becomes a manageable and rewarding endeavour.
Businesses can unlock their data’s full potential by prioritising data quality, transforming it into a strategic asset that drives success and innovation. Contact us today for more information.